When speaking of conversational AI, it is in reference to technologies that users can talk to that includes platforms such as chatbots, virtual agents etc. The access to large volumes of data has made such technologies possible along with the ground-breaking progress in machine learning and natural language processing which facilitates the human interactions that can recognize human language and speech and translates the meanings to produce correct responses in a natural way.
Machine Learning and Natural Language Processing has been the absolute foundations to conversational AI becoming such a widely used and recognised technology platform. Machine learning is a sub-field of artificial intelligence, that uses a vast amount of data to continuously improve using experiences of the world and information around. As more data is inputted as more situations occur the agent becomes more efficient in recognising patterns within the data to then make predictions and making it a useful and relevant platform. This technology combined with the skills of Natural Language Processing, where language is analysed, is what forms conversational AI. NLP has vastly progressed from linguistics to where it is now with the input of machine learning that has made it plausible for conversational AI agents to exist.
In Natural Language Processing there are different steps that generate an appropriate response to the initial input from the user. It begins with the input generation that is provided by the user whether that is through voice or text. There is then an input analysis where the meaning of the input will be determined whether it is via text using Natural Language Understanding (NLU) or if it is a speech input that requires Automatic Speech Recognition (ASR) and then NLU to understand the input. During this process, Natural Language Generation forms a response from historical data. Then finally through machine learning algorithms refine responses overtime to ensure that there is consistent accuracy.
Conversational AI is the next big shift in the world, enhancing customer experience, providing solutions at a much faster rate than waiting on hold to get through to someone. Conversational AI agents are facilitating businesses and providing entertainment purposes as well with personal additions.
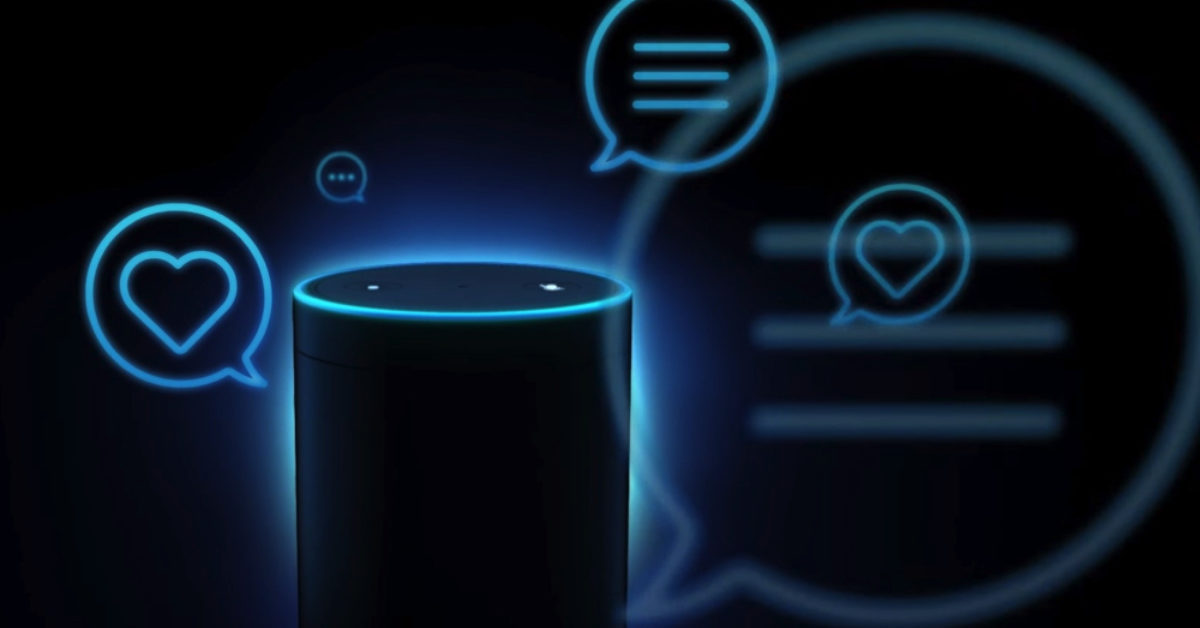
The Rise of Conversational AI
Conversational AI, in apps and on websites, is the next generation of technology, chatbots are evolving to conversational apps. Chatbots are predominantly made up of text and some interactive elements, unlike conversational apps where graphical elements and more are included. The craze of chatbots allowed companies a way to adopt NLP tech to improve user experience and generate more traffic on top of that as well. Chatbots are navigated by predefined flows making them clunky and when there isn’t a predefined flow chatbots began to fizzle out. The use of machine learning technology and NLU combined saw the advancement of conversational AI where such platforms can replace humans in a variety of tasks.
With conversational AI solutions being able to communicate through voice, text, or web it enables such agents to become seamless and responsive to the undetermined conversation. These agents can learn from the history available from other pre-existing conversations and wider data to ensure the agent continues to stay efficient and effective, these agents can then recommend and engage more deeply from this historical data.
The Future of Conversational AI:
Conversational AI as a business is estimated to reach 126 billion U.S dollars by 2025, pushing vast growth in all industries by providing a platform to enhance customer experience. The COVID-19 pandemic accelerated the use of conversational AI agents as a result of dealing with the extreme volume of complaints in a multitude of industries where pre-existing call centres and complaint teams were not at the capacity to handle such volumes, so companies became more dependent upon AI agents. This acceleration has resolved in faster compliant solutions which obviously, in turn, allows an improved customer experience and a likeliness for them to return to the said company if the experience is efficient and dealt with. Within businesses or specific industries, agents can be trained on historical data and the more data inputted the more it learns and the more established it becomes, however with public-facing agents (which are widely used by the population) it can be harder to answer the more general queries and raises more problems when there is less relevant historical data that can be applicable to everyone.
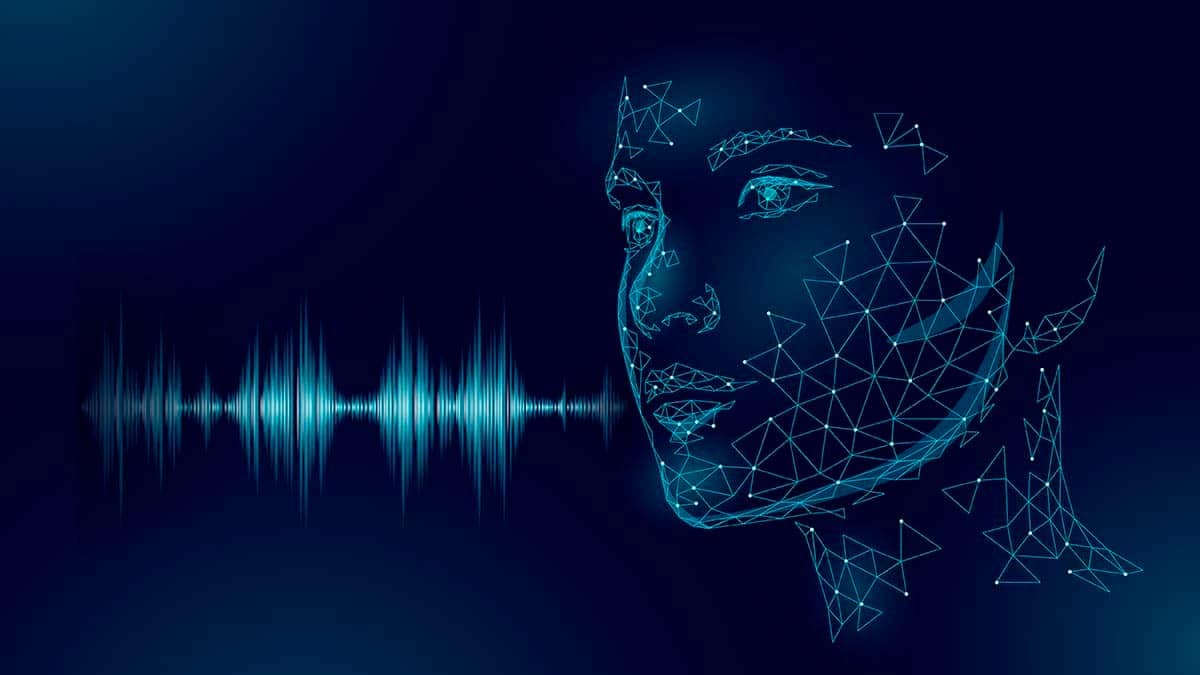
The Issues and Biases in Conversational AI:
One initial problem that conversational AI must deal with begins with the data. It is exceptionally difficult for historical data to be applicable to every single demographic. Different groups, different ages will see issues with varied perspectives. One example of how this works is the way different age groups view privacy, children, and adults view privacy in very different ways. A child has very little consideration for privacy and the information they share compared to an adult and would be more willing to talk about information that their parent would not share. The same applies to ethical issues and different social groups where real-world problems are taken very differently. There is no one fits all ethical standard and principle that applies to each person, yet we all want to have access to the same platform.
This struggle within the conversational AI realm translates into a further problem where there is the challenge of filtering out biases or how to keep out data that is inappropriate, it may not be explicit but certain people may find it offensive. An example of when this problem became visible is when Microsoft launched TAY in 2016 (a Twitter bot) as an experiment in conversational AI. This chatbot engaged publicly on Twitter, learning from the conversations around it and would then tweet according. Within 24hours the chatbot had already been corrupted by the data around and tweeted multiple inappropriate responses.
A solution was formed the year later called Zo and was trained to not discuss politics and religion to avoid engaging in inappropriate conversations. The issue here was that Zo just avoided blacklisted terms rather than assessing the context or linguistics nuances, and even certain countries were blacklisted that had been reported negatively in the news or were commonly associated with crime or atrocities. Human biases and historical biases are exceptionally well documented, and it makes their way into artificial intelligence systems and create harmful results, where the agent can adopt gender stereotypes and racial biases.
Biases make their way into algorithms in several ways where the agents are learning from the data and these biases are currently the source of reducing the potential of AI. Social bias has been a core political problem catching the eyes of the world for years and has now caught the attention of the NLP community where efforts are being made to address the issue of social biases in words and sentences by embedding tasks like sentiment analysis. Another method is addressing fairness and how this can be defined technically where models can define what fairness is in different environments. There are ways AI can meet these standards, where fairness is incorporated into the training process of data beforehand, to counteract social biases.
The solution to social biases in AI and conversational AI is hard to find but with great research being conducted around ways to tackle it, through the input of fairness data and training models to incorporate fairness into the data, there is hope that we can operate with conversational AI without the risk of biases and inappropriate responses.
What do you think? Add your comments below, or get in touch with me at [email protected]!
Get our latest articles and insight straight to your inbox
Hiring Machine Learning Talent?
We engage exceptional humans for companies powered by AI